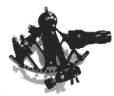
NavList:
A Community Devoted to the Preservation and Practice of Celestial Navigation and Other Methods of Traditional Wayfinding
From: Antoine Couëtte
Date: 2015 Feb 22, 08:59 -0800
RE : Our recent "MPP 2 Parameter Fit" thread by Greg Rudzinski http://fer3.com/arc/m2.aspx/MPP-Two-Parameter-Fit-Rudzinski-feb-2015-g30368
Hello to all,
In recent weeks the use of "MPP 2 and 3 Parameter fit" has been adressed at least twice including within Greg started thread here-above. The second case is a mention of it by one other NavList Member whom I cannot immediately recall.
In case the following topic might not have been adressed earlier on NavList, I would like to submit to your attention, judgement, thoughts and feedback the following "Directions for use" of both MMP "2 Parameter" and "3 Parameter" fits. It is very possible and even probable that this topic has already been adressed somewhere else, but I am not aware of it.
Since examples are worth almost everything, let's use one example from real life.
1 - Let us consider the following set of 4 LOP's and name it "SET#1"
SET#1 #1-1: +3.5 NM / 159° #1-2: +3.0 NM / 171° #1-3: +2.4 NM / 183° #1-4: +1.1 NM / 204°
1.1 - If we process SET#1 through a 2 Parameter fit, we get a "first" Observed Position - let's call it "Position 1.1" - lying into Azimuth 130° at 4.0 NM from DR Position with a Dispersion of Observations = 7.4 E-3 NM (0.0 NM actually)
1.2 - If we further process SET#1 through a 3 Parameter fit, we see that the constant bias on each observed height (e.g. constant index error for example) = +0.15 NM. All observations subsequently reprocessed after removing such +0.15 NM bias yield a "second" position - let's call it "Position 1.2" - lying into Azimuth 128.16° at 3.90 NM from DR Position with a new dispersion value exactly equal to 0.0 NM. So far, so good: we can see that "Position 1.2" remains very close from "Position 1.1" ... STILL we should be already surprised - if not shocked - at the "huge" ratio between the Observation bias (0.15 NM) compared to the Dispersion of Observations (7.4 E-3 NM): this ration exceeds 20 !!!
2 - If we introduce some "random noise" into this same set of observations - only the intercepts are to be modified and not the Azimuths - let's then process the following set of 4 modified LOP's derived from the first set hereabove as follows:
SET#2 #2-1: +2.5 NM / 159° #2-2: +3.5 NM / 171° #2-3: +2.9 NM / 183° #2-4: +0.4 NM / 204°
2.1 - If we process SET#2 through a 2 Parameter fit, we get a "first" Observed Position - let's call it "Position 2.1" - lying into Azimuth 128° at 3.9 NM from DR Position. The Dispersion of Observations = 0.7 NM, a value which remains "fair" and certainly not outrageously high.
Here we can see that "first order" statistics cope quite well with the already significant noise introduced into this second set of observations because "Position 2.1" remains within 1.0 NM from "Position 1.1".
2.2 - If we further process SET#2 through a 3 Parameter fit, we see that the constant bias on each observation (e.g. constant index error for example) = -18.78 NM (over 25 times bigger than the Dispersion of Observations here-above). All observations reprocessed after removing such -18.78 NM bias yield a position - let's call it "Position 2.2" - lying into Azimuth 174.01° at 22.11 NM from DR Position with a new dispersion equal to 0.13 NM. It is no longer possible to "squeeze shrink" the Dispersion value any more given the set of raw data used.
3 - We could study this example in greater depth, and in particular modify SET#1 and SET#2 with one same (known) "constant bias". For example, let's increase each of their intercepts by +1.0 NM to simulate some constant index error.
3.1 - The first order statistics now yield new positions quite close from the early ones unaffected by any previously known "constant Bias", i.e. "Position 1.1" and "Position 2.1".
3.2 - And the higher order statistics (e.g. 3 Parameter MPP) do adequately "smoke out" such (hidden) "constant Bias" and fall back exactly onto the same reprocessed positions, i.e. "Position 1.2" and "Position 2.2".
4 - LESSONS LEARNT:
First Order statistics - e.g. the MPP 2 Parameter fits - remain very robust against noise and even against uncorrected systematic constant error/bias (such as CONSTANT index error) as long as such constant systematic errors remain within some reasonable range: let's say better than 1 arc minute for index error, a quantity which should stay within Navigator's usual reach. In that sense Statistics are GREAT !!!
Higher Order Statistics - e.g. the MPP 3 Parameter fits or equivalent - need to be treated with [much] caution and care. There are a number of cases when they may improve results - especially when processing "high quality" raw data - BUT there are ALSO many cases when they will totally degrade the end-results such as depicted in the example here-above which is not so unfrequent: this example is simply derived from real world morning and noon SUN observations.
Hence the following recommended rules:
First Order Statistics (e.g. 1 Parameter MPP) should be used as frequently as possible.
Care, caution and sound judgment is to be exercised when using Higher Order Statistics (e.g. "3 Parameter MPP"). A good and solid rule of thumb to start trusting LOP's results if and when processed through Higher Order Statistics requires the following 2 conditions:
- Using a sufficient number of observations: 10 to 12 Observations should be such absolute minimum number - AND
- Observations should be evenly distributed over the whole horizon.
Ignorance of these simple 2 rules may hit [quite] hard.
In other words, with Higher Order Statistics, we need to understand what we are doing.
In real life, I very rarely use 3 Parameter MPP fits, but [almost] every single time 2 Parameter MPP fits.
As usual, feedback is welcome :-)
Enjoy !
Kermit
Antoine M. "Kermit" Couëtte