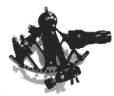
NavList:
A Community Devoted to the Preservation and Practice of Celestial Navigation and Other Methods of Traditional Wayfinding
Re: Is most probable position (MPP) a dangerous misnomer?
From: Bill Lionheart
Date: 2019 Feb 5, 22:22 +0000
From: Bill Lionheart
Date: 2019 Feb 5, 22:22 +0000
I am not a statistician so I only know the bits of statistics I have needed as I go along, but here are a couple of not normal distributions to think about. If you take measurements truncated to a certain number of digits and then average them, this is the average of independent identically distributed uniform variables. The resulting distribution is the Bates Distribution https://en.wikipedia.org/wiki/Bates_distribution. Obviously the central limit theorem tells you it tends towards normal in the limit of averaging infinitely many, but for a small number you see the kurtosis (it is a short tailed distribution) Another two of interest to navigators might be the wrapped normal and von Mises distributions that are used for angles. Obviously an angle cant actually be normally distributed as it wraps around. Maybe the errors in a compass that can spin around wildly if you do some tight turns before settling down, observed by a computer so you cant use common sense to ignore extreme values. Bill On Tue, 5 Feb 2019 at 21:00, Frank Reedwrote: > > Dave, you wrote: > "One little gem which will delight you I’m sure is Anderson’s ‘Is the Gaussian Distribution Normal?’" > > The idea that common measurements ("normal" measurements) are not "normally distributed" (pulled from a Gaussian distribution) is well-known. It's introductory statistics. Folks rediscover this periodically and think they've discovered something quite profound. There are other distributions that one can use. But how can we generate simulated data that broadly resembles real navigational data with its relatively high prevalence of outliers? > > There is a relatively easy way to model the higher prevalence of outliers in sextant observations and other sorts of navigation measurements which allows us to use many of the mathematical properties of Gaussian distributions. We imagine our numbers as being pulled from two bins (both assumed to have mean value equal to zero). One bin, call it bin A, has a relatively "normal" standard deviation, call it s0 (for altitude sights in celestial navigation, s0 might be 0.5 minutes of arc). The other bin, bin B, has a higher standard deviation, call it s1. And s1 might be, in a typical real-world modelling case, three times larger than s0. Number are drawn from bin A some large fraction of the time, e.g. 80% of instances, and drawn from bin B the rest of the time. Of course the numbers aren't labeled with the bin that they came from so all you get in the end is a bunch of numbers with some statistical properties. Those numbers will have a net standard deviation somewhat greater than s0, but the key property is that they, collectively, will not correspond to a Gaussian distribution because there will be more "outliers". The "tails" of the distribution are thicker, more heavily-populated. A statistical measure of this is known by the rather ugly, jargon-y term "kurtosity". > > So if you're simulating observations, don't use a simpleGaussian. Use a pair of Gaussians, as above. This is a nice, easily-implemented technique for generating model data for navigation simulations, and it can help find cases where the "normal" math might lead you astray. > > Frank Reed > >